The Fallacies of Models
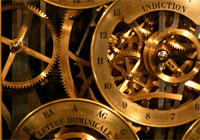
Questions such as what's happening to our climate, which direction the markets will move and where trust comes from, appear to be so complex they seem unanswerable.
One way to get our heads round these questions is to make a model. “Modelling is really important to allow scientists and engineers to be able to understand what's going on and then hopefully predict what's going to happen in the future,” says Roy Kalawsky, Professor of Systems Engineering at Loughborough University. But when it comes to models there a few things to be wary of.
The Three Fallacies
Models are made with simplifications and assumptions about the real world. Some aspects are discounted as insignificant while others make it in. “[The model] can never be the real thing because there are things you haven't taken into account in the modelling process that could have a tiny but still important impact later on,” says Roy. And this leads to our first fallacy: the fallacy of missing what's important.
The second fallacy is about understanding who's going to use your model: the fallacy of interpretation. “Understanding who's going to use it is very important," says Roy. A model of a bridge for a structural engineer is going to be different from a model for a council worker looking at traffic flow.
The final fallacy is about testing.
“One of biggest challenges I feel in the modelling process is being able to validate and verify the model you've created," says Roy, "The modelling process doesn't finish when you've made the model, because you've got to compare the outputs from the model or what you observe in the model against what you can see in the real world. If it doesn’t comply then you have to try and understand why that is so you go back and refine the model."
But for some situations, there just aren’t many recorded examples to compare against-the spread of a virus or global pandemic, for example. So while you can make a model there are sometimes so few occurrences it's almost impossible to know whether your model reflects real life.
Social modelling
Modelling social interactions is particularly hard. How do you get inside someone's head to know what they are thinking?
Researchers have plenty of ideas about how people think – just look at the Wikipedia list of philosophies – but there are no clear answers, and often just more questions. For example, economic frameworks suggest people make decisions to maximise benefits to themselves. But we know people don’t always behave like selfishly, so where does altruism come from? One way to tackle these types of questions is to use a technique called agent based modelling. “We represent people and their interactions individually. You set up a world with micro rules on how you think people behave, interact and communicate with each other in this circumstance and you set them going and see what happens and the social structures, issues and tendencies that emerge,” says Bruce Edmonds, Director of the Centre for Policy Modelling at Manchester Metropolitan University.
Here the world is inside a computer programme filled with hundreds and thousands of little robots who make decisions based on a set of rules they are programmed with. These rules are based on data gathered by social scientists. “Some people approach the problem with some algorithmic process that they think captures some aspect or relevant aspect of how people think. We complement that by looking at data mining or qualitative evidence about how people behave, so a lot of social scientists interview people and produce very rich but very context specific descriptions of how people behave. We try and take those and provide a menu of possibilities of how people behave and try to look at data to try and decide which of those sorts of behaviours are more frequent,” says Bruce.
The models can’t predict how probable an event is, but they do reveal the range of possible outcomes.
But even these agent based models can still succumb to the fallacies, “It is very easy to be given a false sense of security with these models. A real problem in social science is getting enough data. You have a little bit of observation here, bit of social psychology here, a survey about a different number of people here, a disconnect time series, and you never really get them about the same people. So you don't get rich enough data to pin down what are inevitably somewhat more complex models,” says Bruce.
Here come the physicists
But new interdisciplinary teams are starting to mix social scientists with physicists. Physicists bring an ability to condense the data that social scientists have discovered through interviews, surveys or observation into a rigorous, testable model. The models need to have adequate complexity to give credible outputs but not so complex they don’t function properly.
Physicists tap into their library of mathematical techniques to understand and characterise the interactions shown within the data. And in doing so try to distill what factors are important or if there are any approximations that can be made.
It’s a hard balancing act. “Physicist and the way they think about problems are so different to the way social scientists think about problems,” says Bruce, “and we’re still in the middle of testing whether physics can help us narrow down these problems.”
But even though new modelling techniques are emerging it's important to keep in mind that all models can fall to any of the three fallacies.
Further reading
The Conversation - Economics and the brain: how people really make decisions in turbulent times
Wired - Mathematical Model for Surviving a Zombie Attack
New York Times - A Technological Edge on Wildfires
Ars Technica - Why trust climate models? It’s a matter of simple science
io9 - Bonini's Paradox proves that you can't make a useful AND accurate model
Top image credit: Flickr/frans16611
Share:
The folowing links are external